Estimation non-paramétrique univariée#
Objectif
modéliser les fonctions de survie et de risque avec Kaplan-Meier & Nelson-Aalen
étudier l’impact de la censure sur les estimateurs
étudier l’impact d’une vidéo promotionnelle sur les estimateurs
Import des outils / jeu de données#
1import pandas as pd
2
3from src.modelisation.univariate.non_parametric.models import (
4 create_hazard_models,
5 create_survival_models,
6)
7from src.modelisation.univariate.parametric.plot import (
8 plot_hazard_estimation,
9 plot_survival_estimation,
10)
11from src.utils import init_notebook
1init_notebook()
Données#
1df = pd.read_csv(
2 "data/kickstarter_1.csv",
3 parse_dates=True,
4)
1event_times = df["day_succ"]
2event_observed = df["Status"]
3
4event_times_no_censoring = df["day_succ"][df["Status"] == 1]
5event_observed_no_censoring = df["Status"][df["Status"] == 1]
1df_video = df[df["has_video"] == 1].copy()
2df_no_video = df[df["has_video"] == 0].copy()
3
4t_video = df_video["day_succ"]
5o_video = df_video["Status"]
6
7t_no_video = df_no_video["day_succ"]
8o_no_video = df_no_video["Status"]
Fonction de survie#
1survival_models = create_survival_models()
Kaplan-Meier#
Formalisation du problème#
Soit \(\tau\) une variable aléatoire à valeur dans \(\mathbb{N}^*\) modélisant le jour de succès d’un projet Kickstarter.
On se munit d’un échantillon \(\tau_1,\dots,\tau_n\) de variables aléatoires indépendantes identiquement distribuées suivant la même loi que \(\tau\) et de
\((c_1, \dots, c_n)\) le vecteur déterministe des instants de censure tel que
Pour construire l’estimateur de Kaplan-Meier et afin de prendre en compte la censure, on s’intéresse aux couples d’observations \(( \tilde \tau_j, c_j )_{j=1,\dots,n}\)
où \(\tilde \tau_j = \min(\tau_j,c_j)\) pour tout \(j \in [1 ; n]\).
Calcul de l’estimateur de Kaplan-Meier#
Fonction du survie#
Soit un jour \(t \in \mathbb{N}\), la fonction du survie pour \(t\) vaut :
où \(q(t) = 1-\operatorname{Prob}(\tau=t\mid\tau\ge t)\).
Par itération on obtient $\( S(t) = q(t) q(t-1) \cdots q(0). \)$
Construction d’un estimateur de \(q(t)\)#
Soit \(t \in \mathbb{N}\)
De plus, on a les égalités suivantes : $\( \operatorname{Prob}(\tau=s) = \operatorname{Prob}(\tilde \tau_k=s) \)\( \)\( \operatorname{Prob}(\tau\ge s) = \operatorname{Prob}(\tilde \tau_k\ge s) \)$
Un estimateur de \(q(t)\) est alors donné par :
où \(d(t)\) est le nombre de succès connus au temps \(t\)
et \(n(t)\) est le nombre de projets qui n’ont pas encore réussi et non censurés au temps \(t-1\).
Retour au calcul de l’estimateur de Kaplan-Meier#
Enfin, on a l’estimateur de Kaplan-Meier pour la fonction de survie avec la censure : $\( \hat S(t) = \prod_{i:t_i\le t} \left(1-\frac{d_i}{n_i}\right) \)$
Propriétés statistiques de l’estimateur de Kaplan-Meier#
Biais#
Estimateur à biais positif : \(bias[\hat S(t)] = \mathbb{E}[\hat S(t)]- S(t) >= 0\)
Estimateur asymptotiquement sans biais : \(\displaystyle \lim_{n \to +\infty} \hat S(t) = 0\)
Variance#
Une façon courante de calculer la variance est la formule de Greenwood : $\( \widehat{\operatorname{Var}} \left( \widehat S(t) \right) = \widehat S(t)^2 \sum_{i:\ t_i\le t} \frac{d_i}{n_i(n_i-d_i)} \hspace{12px} \forall t \in \mathbb{N} \)$
1plot_survival_estimation(
2 survival_models["Kaplan-Meier"], event_times, event_observed, "Kaplan-Meier"
3)
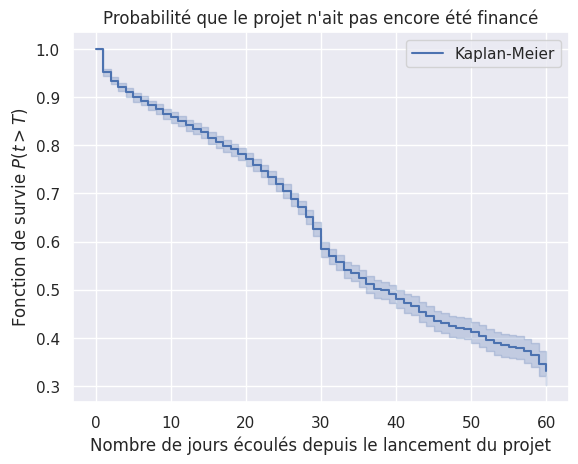
Kaplan-Meier (avec et sans censure)#
1plot_survival_estimation(
2 survival_models["Kaplan-Meier"], event_times, event_observed, "avec censure"
3)
4plot_survival_estimation(
5 survival_models["Kaplan-Meier"],
6 event_times_no_censoring,
7 event_observed_no_censoring,
8 "sans censure",
9)
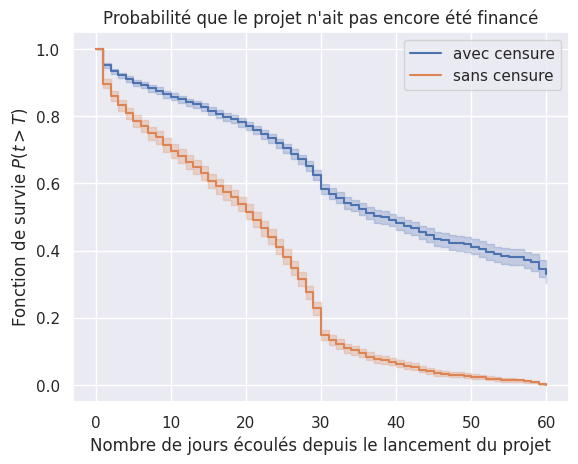
Co-variables#
Vidéo de présentation#
1plot_survival_estimation(
2 survival_models["Kaplan-Meier"], event_times, event_observed, "population complète"
3)
4plot_survival_estimation(
5 survival_models["Kaplan-Meier"], t_video, o_video, "avec vidéo"
6)
7plot_survival_estimation(
8 survival_models["Kaplan-Meier"], t_no_video, o_no_video, "sans vidéo"
9)
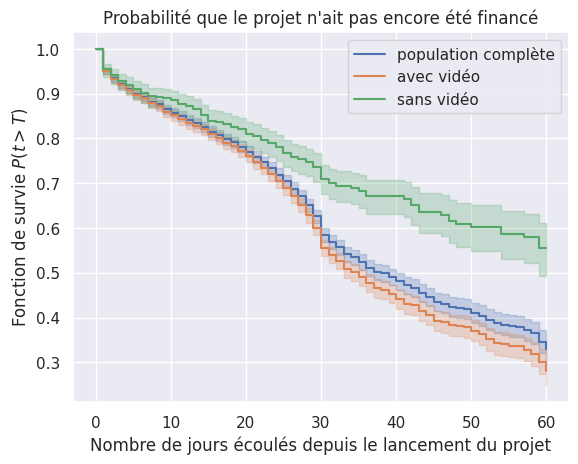
Fonction de risque#
1hazard_models = create_hazard_models()
Nelson-Aalen#
1plot_hazard_estimation(
2 hazard_models["Nelson-Aalen"], event_times, event_observed, "Nelson-Aalen"
3)
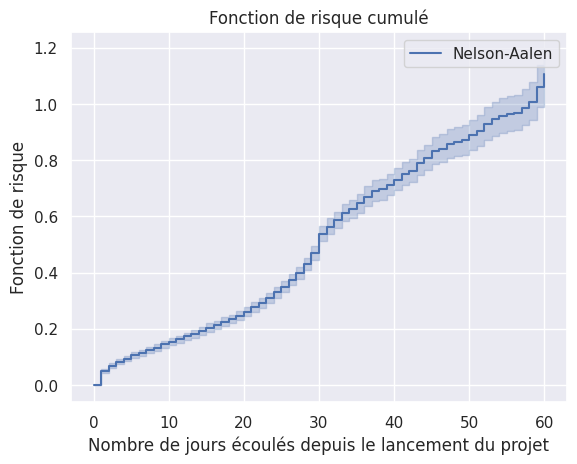
Nelson-Aalen (avec et sans censure)#
1plot_hazard_estimation(
2 hazard_models["Nelson-Aalen"], event_times, event_observed, "avec censure"
3)
4plot_hazard_estimation(
5 hazard_models["Nelson-Aalen"],
6 event_times_no_censoring,
7 event_observed_no_censoring,
8 "sans censure",
9)
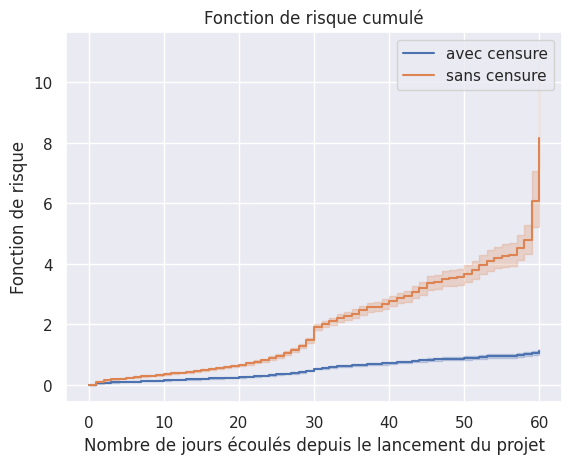
Co-variables#
Vidéo de présentation#
1plot_hazard_estimation(
2 hazard_models["Nelson-Aalen"], event_times, event_observed, "population complète"
3)
4plot_hazard_estimation(hazard_models["Nelson-Aalen"], t_video, o_video, "avec vidéo")
5plot_hazard_estimation(
6 hazard_models["Nelson-Aalen"], t_no_video, o_no_video, "sans vidéo"
7)
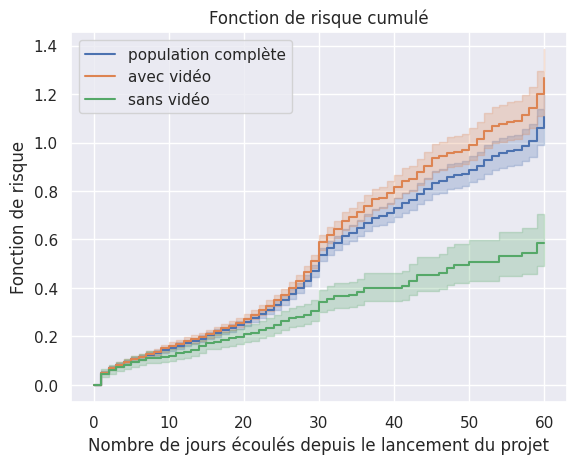